phmhaven: Your Guide to Prognostics and Health Management
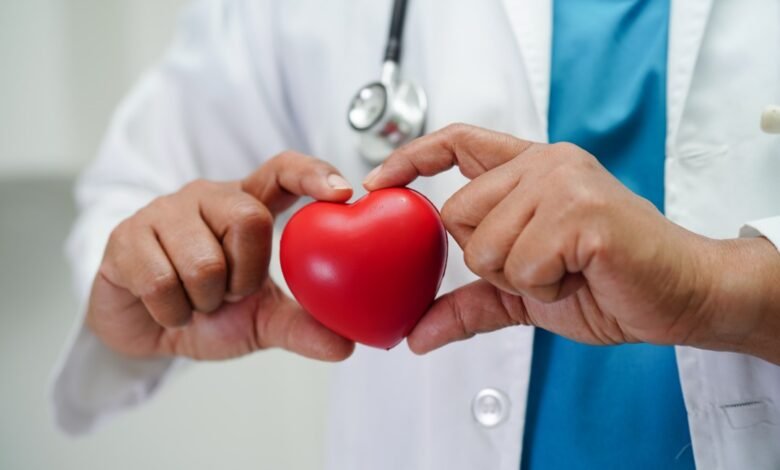
In an increasingly interconnected world, where everything from airplanes to industrial machinery relies on sophisticated technology, maintaining the health and functionality of these systems has never been more critical. Enter Prognostics and Health Management (PHM) — a field that blends data, analytics, and engineering to monitor, predict, and manage the health of complex systems. “phmhaven” is where these innovative concepts converge to help industries make informed, proactive decisions that save costs, enhance reliability, and optimize performance.
In this article, we’ll dive into what phmhaven is, how it impacts industries, its core principles, and where it’s headed in the future.
1. Introduction to Prognostics and Health Management (PHM)
Prognostics and Health Management (PHM) is a field that focuses on predicting the future health and functionality of a system, allowing for timely intervention before issues arise. The goal is not only to repair but to prevent failures from happening in the first place. By integrating data analytics, machine learning, and engineering principles, PHM provides actionable insights to keep systems running smoothly.
PHM has become crucial for industries like aviation, automotive, manufacturing, and healthcare. Imagine if an aircraft could alert its engineers about a potential issue before it even takes off. Or if a hospital could monitor its critical equipment to prevent downtime during a critical operation. PHM is making this vision a reality.
Why Prognostics and Health Management?
The need for PHM arises from the demand for more reliable and cost-effective maintenance strategies. Traditional maintenance approaches, like reactive or preventive maintenance, are either inefficient or costly. PHM, in contrast, uses data and predictive algorithms to perform Condition-Based Maintenance (CBM), where interventions happen only when needed, and predictions are made based on the system’s actual condition.
2. What is phmhaven?
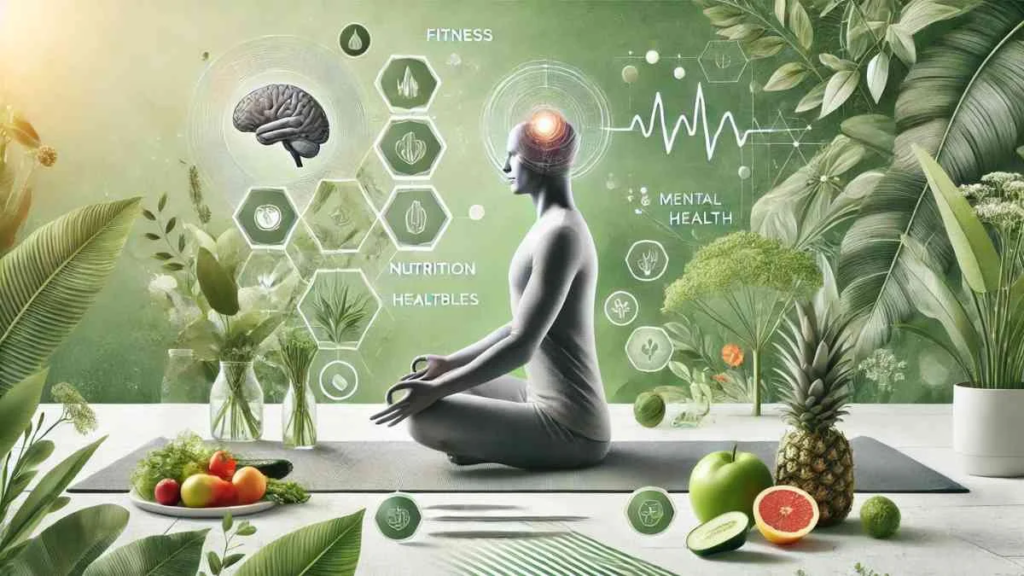
“phmhaven” represents a hub or platform dedicated to everything related to Prognostics and Health Management. This term encapsulates not only the tools and techniques used in PHM but also the community, resources, and knowledge-sharing opportunities available for professionals, researchers, and enthusiasts in the field.
phmhaven is an ecosystem designed to support individuals and organizations in understanding and implementing PHM strategies. This ecosystem includes:
- Educational Resources: Tutorials, guides, and courses to learn about PHM concepts.
- Data Repositories: Access to datasets essential for training models in PHM applications.
- Tools and Software: Specialized software and toolkits designed for PHM tasks.
- Community Forums and Networking: A place for professionals and academics to connect, discuss, and collaborate.
phmhaven thus serves as a comprehensive resource center, bringing together the components, technology, and community necessary to advance the field.
3. Core Components of phmhaven
phmhaven is built on a few fundamental pillars, each crucial to its function and value. Understanding these core components helps to appreciate how phmhaven integrates various aspects of prognostics and health management.
3.1 Monitoring
Monitoring is the first step in PHM, involving continuous observation of a system’s performance. Sensors and IoT devices play a critical role here, collecting real-time data on parameters like temperature, vibration, pressure, and more.
3.2 Diagnostics
Diagnostics analyzes data to determine if there is an existing fault or anomaly in the system. This step helps identify the root cause of a problem, enabling quicker and more accurate corrective actions.
3.3 Prognostics
Prognostics, the heart of PHM, involves predicting when a system or component is likely to fail based on current and historical data. Various machine learning and AI techniques are applied to forecast the Remaining Useful Life (RUL) of a system, providing a timeline for potential maintenance.
3.4 Health Management
Health management takes the insights from monitoring, diagnostics, and prognostics and translates them into actionable decisions. This can mean scheduling maintenance, adjusting operational parameters, or implementing contingency plans to avoid failures.
3.5 Data Processing and Analysis
Raw data from sensors is often noisy or incomplete, requiring data cleaning and preprocessing before it can be used. phmhaven provides tools and resources for data preprocessing, including anomaly detection, feature extraction, and filtering techniques.
4. The Role of Data in PHM
Data is at the core of PHM, and it’s what makes the predictions and insights of phmhaven possible. The effectiveness of any PHM system largely depends on the quality and quantity of data collected. Here’s how data contributes to PHM:
- Data Collection: Sensors collect raw data in real time from equipment and machinery.
- Data Preprocessing: Data cleaning, filtering, and normalization improve the quality of data for better analysis.
- Data Analysis: Advanced algorithms analyze the data to detect anomalies and patterns.
- Predictive Modeling: Machine learning models are trained on historical data to predict future states of health.
phmhaven not only stores vast amounts of data but also provides frameworks and tools to analyze it. This data-driven approach empowers organizations to base their decisions on concrete, actionable insights rather than on assumptions or educated guesses.
5. PHM Methods and Techniques
PHM employs several methods and techniques to achieve its goals. Here are some of the primary methods:
5.1 Machine Learning and AI
Machine learning algorithms such as decision trees, neural networks, and support vector machines are popular for building predictive models in PHM. Deep learning and reinforcement learning techniques are also gaining traction, especially in systems with complex, nonlinear behaviors.
5.2 Statistical Methods
Statistical methods, such as regression analysis and Bayesian networks, help model the relationships between different system parameters and predict potential issues. These techniques are crucial when dealing with probabilistic failures.
5.3 Signal Processing
Signal processing techniques filter out noise and extract meaningful features from sensor data. Techniques such as Fourier transforms and wavelet analysis allow for precise analysis of vibration, acoustic, and other sensor signals, which are critical in many industrial PHM applications.
5.4 Physics-Based Models
In some cases, systems are modeled based on physics and engineering principles. For example, physics-based models can simulate wear and tear in mechanical systems, enabling more accurate predictions of component life.
5.5 Hybrid Approaches
Hybrid approaches combine machine learning, statistical models, and physics-based models. This approach allows PHM systems to leverage the strengths of each method, providing a more robust and accurate solution.
6. Applications of phmhaven in Various Industries
PHM’s versatility makes it valuable across multiple sectors. Here’s how phmhaven is being applied in various fields:
6.1 Aerospace and Aviation
In the aviation industry, reliability is paramount. phmhaven supports condition-based maintenance for aircraft, reducing downtime and improving safety. Predictive models can alert engineers of potential issues, ensuring that aircraft stay in peak condition.
6.2 Manufacturing
Manufacturing involves complex machinery and processes that need to run continuously. phmhaven enables predictive maintenance in factories, preventing unexpected equipment failures and maximizing productivity.
6.3 Automotive
The automotive industry leverages PHM to monitor vehicles and provide drivers with maintenance alerts. For instance, many electric vehicles use PHM to track battery health and longevity.
6.4 Energy and Utilities
For power plants and renewable energy systems, phmhaven ensures that equipment remains operational without unexpected breakdowns. In wind and solar energy, PHM is vital to monitor and maintain remote equipment effectively.
6.5 Healthcare
PHM’s applications in healthcare are also promising. For example, phmhaven can be used to monitor critical medical devices, ensuring they remain reliable, especially during emergencies.
7. Benefits of PHM Haven
The advantages of implementing phmhaven in any organization are substantial:
- Increased Reliability: PHM ensures that systems remain operational for longer, reducing the chances of unexpected failures.
- Cost Savings: By preventing costly repairs and downtime, PHM helps organizations save on maintenance and operational costs.
- Improved Safety: With predictive maintenance, PHM improves safety by addressing potential risks before they cause harm.
- Resource Optimization: PHM enables better resource allocation, as systems are only maintained when necessary.
8. Challenges and Limitations
While PHM offers immense benefits, implementing it is not without challenges:
- Data Quality and Availability: Poor data quality can lead to inaccurate predictions, making data integrity a primary concern.
- High Initial Investment: Setting up PHM systems, especially for large-scale operations, can be costly.
- Technical Expertise: Implementing PHM requires skilled professionals in data science, machine learning, and engineering.
- Complexity of Systems: For highly complex systems, creating accurate models and predictions can be challenging.
9. Future Trends in PHM and phmhaven
The future of PHM and PHM Haven looks bright, with new advancements and technologies on the horizon:
9.1 Integration with IoT
As more devices connect to the Internet of Things (IoT), PHM will become more accessible and effective, with IoT-enabled devices continuously feeding data to PHM systems.
9.2 Artificial Intelligence Advancements
AI is expected to play a significant role in the future of PHM, with improved algorithms for anomaly detection, predictive modeling, and decision-making.
9.3 Edge Computing
Edge computing allows data to be processed closer to where it’s generated, reducing latency and making PHM systems faster and more efficient.
9.4 Digital Twins
A digital twin is a virtual representation of a physical system that allows real-time monitoring and simulation. In the future, digital twins will become standard in PHM Haven, providing an even more comprehensive view of system health.
10. Conclusion
PHM Haven represents a powerful convergence of technology, data, and expertise, providing organizations with the tools they need to anticipate, prevent, and manage system failures. By focusing on predictive and proactive maintenance, PHM Haven not only optimizes performance but also contributes to sustainability, safety, and cost-effectiveness in numerous industries.
As technology advances, PHM Haven will likely grow in capability and accessibility, enabling even more organizations to harness the benefits of a proactive approach to system health. The journey of PHM has only begun, and the future holds exciting possibilities for making our world safer, more efficient, and more resilient.